Der Award
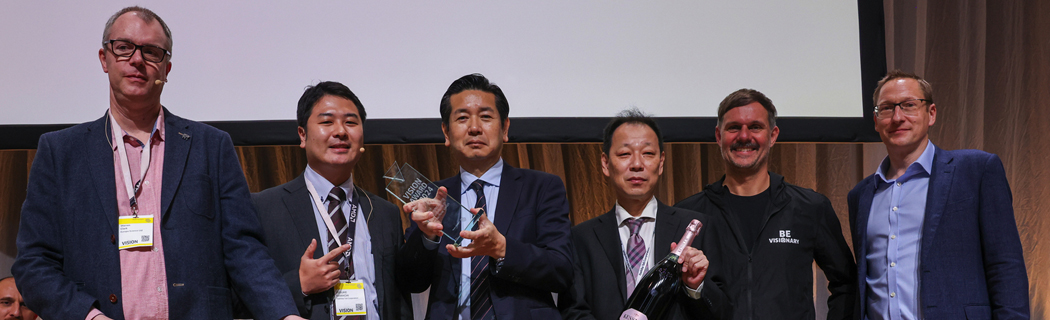
Der begehrte VISION Award wird traditionell zur VISION verliehen. Mit der renommierten Auszeichnung prämiert eine hochkarätige Jury die beste Innovation aus dem Bereich der Bildverarbeitung. Die Auszeichnung ist mit einem Preisgeld von 3.000 Euro dotiert, welches die britische Zeitschrift Imaging and Machine Vision Europe (IMVE) ausgelobt hat.
Im Frühjahr 2026 öffnen wir die Plattform für alle interessierten Firmen, um ihre Innovationen einzureichen.
Rückblick VISION 2024
Die Jury 2024
|